For nearly 100 years, scientists have mused over what dictates the crystal structures of solids. Researchers at Howard University recently used National Science Foundation ACCESS allocations on three supercomputers to better understand the intricate details of an important class of two-dimensional layered materials called transition metal dichalcogenides, or TMDs. Scientifically these materials are appealing for fundamental studies because of the unique properties of different TMDs, but also because they show promise for a number of applications – from flexible electronics and spintronics to optoelectronics and memory devices.
While fundamental materials research like this has traditionally employed experiments and/or theoretical calculations, machine learning allowed the researchers to take a novel look at hidden factors dictating structural preferences of TMDs.
Simulations generated on Comet and Expanse at the San Diego Supercomputer Center (SDSC) at UC San Diego and Bridges and Bridges-2 at the Pittsburgh Supercomputing Center (PSC) revealed the most important factors that dictate the crystal structures (phases) of TMDs. The researchers, led by Physics Professor Pratibha Dev, Postdoctoral Researcher Pankaj Kumar and Research Scientist Sharmila Shirodkar (Howard University), as well as Vinit Sharma (Oak Ridge National Laboratory, or ORNL), recently published their findings in a paper entitled “Predicting phase preferences of two-dimensional transition metal dichalcogenides using machine learning” in Physical Review Materials.
Since we do not have access to computer clusters at Howard, most of our resource-intensive quantum mechanical calculations are performed on supercomputers provided via XSEDE/ACCESS infrastructure. Hence, without ACCESS/XSEDE resources, this research would not have happened.
Pratibha Dev, physics professor, Howard University
Dev explained that her research group at Howard University has been studying TMDs for several years to determine their phase change properties so that they’re useful in memory devices. This led them to their interest in combining quantum mechanical calculations along with machine learning analyses to closely examine phase preferences of TMDs. The team’s machine learning model not only rediscovered known physicochemical attributes considered by earlier researchers, but also discovered additional factors that were previously unknown to influence structural preferences displayed by TMDs.

“Our work combines more traditional theoretical tools that are used to study materials’ properties with machine-learning techniques in order to address a six-decade-old question about TMDs,” Dev said. “TMDs are three-atom-thick layers that tend to adopt one of several structures – or phases and the properties are dictated by the structure a TMD adopts. This gives rise to an important question: What dictates the observed structural preferences of TMDs?”
Dev noted the Howard and ORNL team’s work on ACCESS resources demonstrates how machine learning can be used to tackle old problems in condensed matter physics. Specifically, by determining underlying factors that dictate the structural preferences of TMDs, this work helps to understand – and how to best manipulate – composition-structure-property relationships.
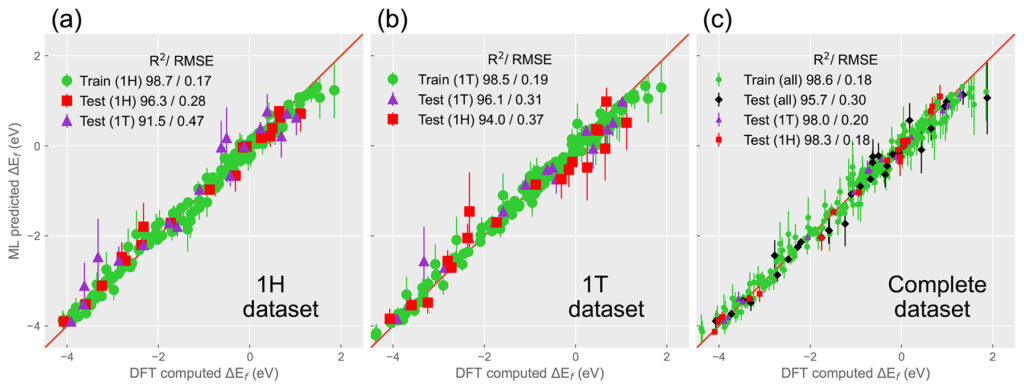
“This paves the way for engineering properties of TMDs and an array of applications,” she said. “We did not expect to find a better predictor of structure than the relative values of radii – expressed either as a ratio or a difference – however, our simulations on SDSC and PSC resources revealed that the relative values of dipole polarizabilities of the cations and anions is an even better predictor of structural preference than the radii.”
“We were especially appreciative of the collaboration with the Extended Collaborative Support Service (ECSS) program and specifically Vinit Sharma at ORNL, who participated in our machine learning efforts,” she continued. “Our work allowed us to develop a framework that is generalizable and provides a path forward in materials research.”
More information on obtaining an ACCESS allocation can be found here.
Project Details
Resource Provider Institution: San Diego Supercomputing Center (SDSC), Pittsburgh Supercomputing Center (PSC)
Affiliations: Howard University, Oak Ridge National Laboratory
Funding Agency: NSF
Grant or Allocation Number(s): PHY180014 and TG-DMR200008
The science story featured here was enabled by the ACCESS program, which is supported by National Science Foundation grants #2138259, #2138286, #2138307, #2137603, and #2138296.