Detailed satellite images of the Arctic are always incredibly interesting, yet the methods of collecting, sorting and analyzing them are no small feat. While a company called Maxar Technologies has been taking and collecting satellite images of the Arctic multiple times a year for several years, the data is large and simply too much for a typical computer to sort and analyze. ACCESS allocations on Delta at the National Center for Supercomputing Applications (NCSA) at University of Illinois Urbana Champaign (UIUC) were recently used by researchers at the University of Connecticut to determine how this imagery could be easily sorted and analyzed by teams studying the region.
Specifically, Chandi Witharana, an assistant professor of natural resources and the environment at the University of Connecticut, co-authored a paper entitled A multi-objective comparison of CNN architectures in Arctic human-built infrastructure mapping from sub-meter resolution satellite imagery in the International Journal of Remote Sensing that details the team’s most recent findings.
Witharana said that the team looked at ways that large sets of detailed images are used by Arctic researchers – ranging from scientists studying changes in the ground caused by permafrost to the impact of structures.
“Understanding the risks to infrastructure in ice-rich areas due to thawing permafrost is crucial for adapting to climate change in the Arctic; it’s also important no matter what the climate is doing because infrastructure increases risks to permafrost thaw,” Witharana said. “But to do this, we need detailed maps of buildings and other structures, which are often lacking, so our study used ACCESS allocations on Delta to make mapping efforts less costly and more efficient.”
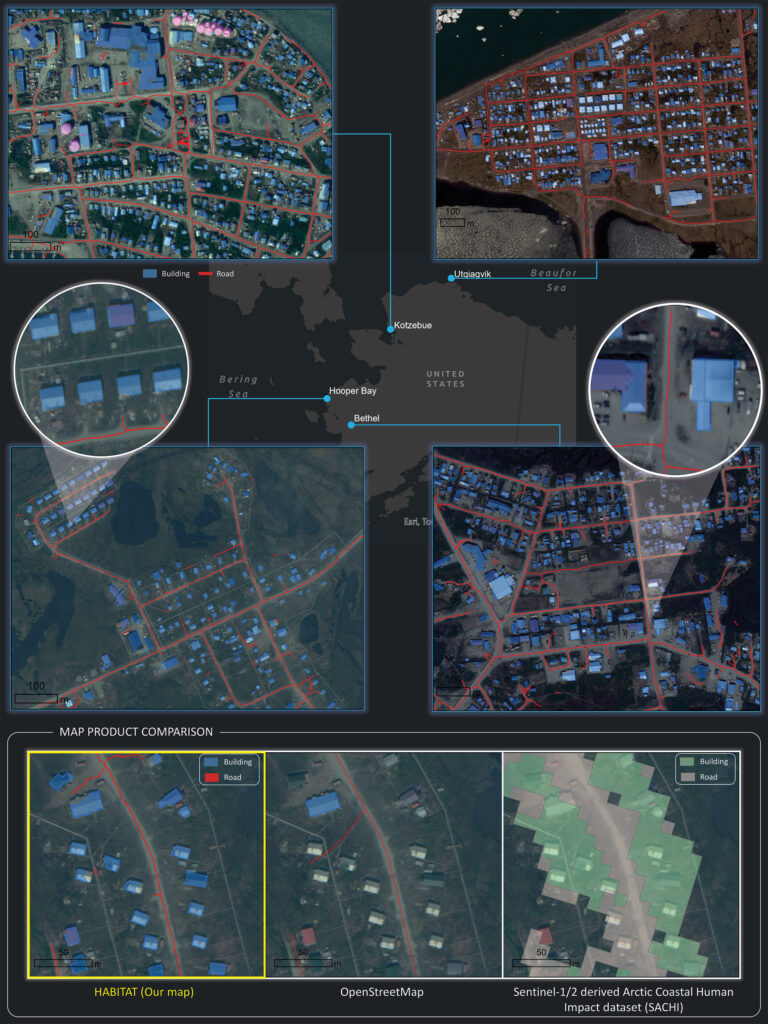
The study laid the foundation for building an artificial intelligence (AI) model that can automatically detect various kinds of human-built structures from high-resolution satellite imagery of Arctic communities. Using a dataset containing sub-meter-resolution satellite images and corresponding outlines of infrastructure (e.g., houses, roads, pipelines, cisterns etc.) of communities across the Arctic, the team trained and tested nine different convolutional neural networks (CNNs) – deep-learning models that allow computers to detect objects and categories in images by learning to recognize and understand visual patterns in the same way humans do – to determine which would be the most accurate and efficient model in preparation for a future pan-Arctic application.
By splitting the dataset several ways using a statistical resampling technique known as cross-validation, they evaluated each model 10 times and used statistical significance testing to determine from these samples if one model performed better than the others. The team found that one particular CNN model, ResNet-50-UNet++, outperformed the others while requiring comparatively fewer computational resources to run on the Delta supercomputer.
“We trained and tested close to 100 different deep-learning models to gather an adequate number of samples for statistical testing, much of which occurred simultaneously as we could submit multiple jobs at once,” explained Elias Manos, a graduate student at Connecticut and lead author on the paper. “Without ACCESS allocations on the Delta supercomputer at NCSA, this work would likely not have been possible.”
Manos said that since they’ve now found a deep learning model that performs well in infrastructure detection, they can further tune the model and eventually apply it to even larger amounts of high-resolution satellite imagery that cover all communities across the Arctic.
“This will be monumental for scientific discovery among Arctic scientists and governments that need to understand the risk that thawing permafrost poses to the infrastructure it is built upon,” Manos said. “Many structures in the Arctic sit on top of foundations that rely on the stability and strength of frozen ground, which is problematically thawing out due to rapid climate change.”
While there are maps of buildings and roads across the Arctic – including Alaska, Canada and Russia – many regions are still unaccounted for due to a lack of attention given to remote areas of the Arctic. The team’s ultimate goal is to produce the first pan-Arctic map of various types of infrastructure at a sub-meter spatial resolution and make it publicly accessible.
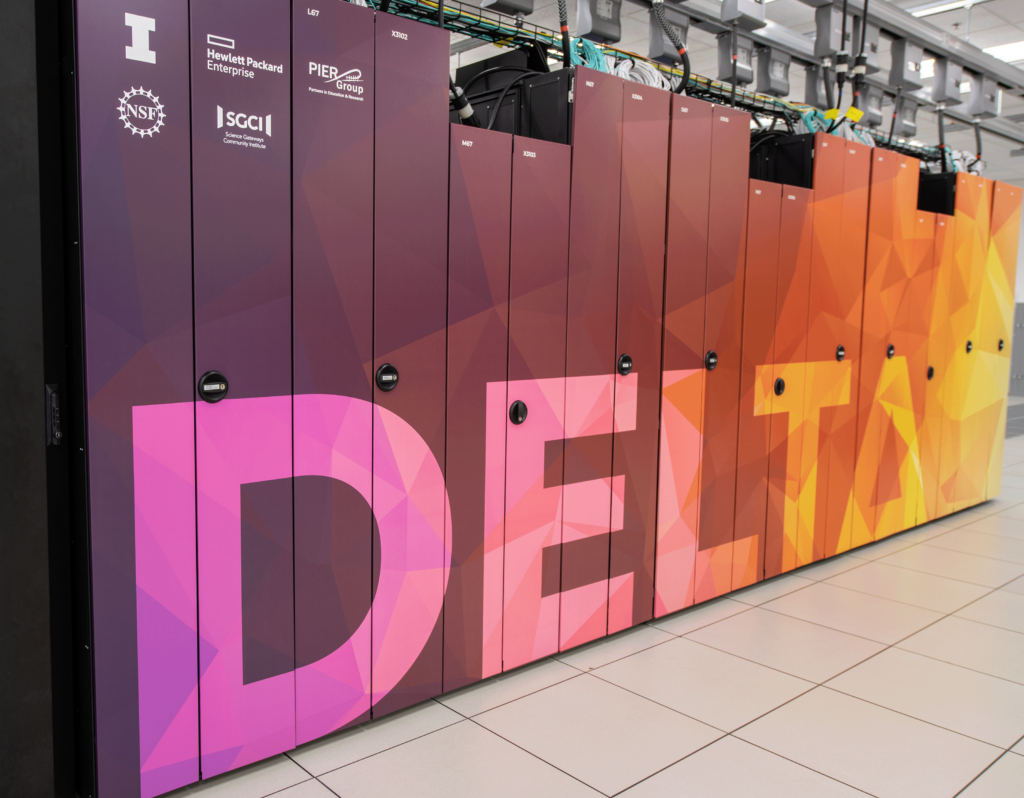
We trained and tested close to 100 different deep-learning models to gather an adequate number of samples for statistical testing, much of which occurred simultaneously as we could submit multiple jobs at once. Without ACCESS allocations on the Delta supercomputer at NCSA, this work would likely not have been possible.
–Elias Manos, graduate student, University of Connecticut
“To run our model on the imagery of every human settlement built on permafrost within the circumpolar Arctic, we will heavily rely on ACCESS allocations so that we can continue our work on Delta,” Witharana said.
Witharana said to create and share the imagery-derived geospatial maps, the team works with Anna Liljedahl, an associate scientist at the Woodwell Climate Research Center. Liljedahl, also co-author of the paper, is leading the Permafrost Discovery Gateway effort, which aims to make big geospatial data accessible to anyone with an internet connection.
“Creating useful big geospatial data is step one; step two is to support knowledge generation from that information; how we’ve been doing and delivering science in the past is not helpful in either creating or sharing big geospatial data,” Liljedahl said. “We need to go beyond old methods and communication paths and enable science to reach people more timely and in more accessible ways.”
She explained that the gateway will hold an array of tools that allow researchers, educators and the general public to access imagery-derived product related to the Arctic – including those sorted and analyzed by Witharana and Manos.
“Manos’ infrastructure product makes all the environmental datasets much more exciting as it adds the human dimension,” Liljedahl said.
“Arctic imagery work like this is exactly why advanced research computing and data resources and service available via ACCESS are important assets to the U.S. research community,” said John Towns, principal investigator for the ACCESS Coordination Office. “We are excited to see where this work goes and encourage collaborations with researchers as they address their big data challenges.”
Project Details
Resource Provider Institution(s): National Center for Supercomputing Applications (NCSA)
Affiliations: University of Connecticut, Woodwell Climate Research Center, Maxar Technologies
Funding Agency: This research was funded by the National Science Foundation, Navigating the New Arctic program (grant nos. 1927723, 1927872 and 2052107).
Grant or Allocation Number(s): DPP190001 and EES220055
The science story featured here was enabled by the U.S. National Science Foundation’s ACCESS program, which is supported by National Science Foundation grants #2138259, #2138286, #2138307, #2137603, and #2138296.